- I wanted to contol the CBiS micro:Bit Car via gestures whilst holding another micro:Bit (see Figure 1) I went for: - Button A in combinat...
- You don't need to buy a robot to get programming a robot, now there are a range of free and relatively simple to start with robot simula...
- With the sad news that Anki is shutting down ( https://www.vox.com/2019/4/29/18522966/anki-robot-cozmo-staff-layoffs-robotics-toys-boris-sof...
- For a few years, I have been a fan of Aframe and AR.js - these are fantastic tools for creating web-based Virtual and Augmented Reality. No...
- The Raspberry Pi Foundation recently released a programming activity Alien Language , with support Dale from Machine Learning for Kids , tha...
- For a number of years, I have been playing with robots as a means of developing programming/coding skills with students. The problem is when...
- It is not physical but CBiS Education have release a free robot arm simulator for Scratch. Downloadable from their site http://w...
- I recently tried out a new (well new to me) robot Codey Rocky - and yes I am going to tell people it's name is Rocky- for STEM outreach...
- I am going to try to persuade you that using A-Frame it is not hard to do some simple Augmented Reality (AR) for free, via a browser, but th...
- The microbit is a great piece of kit, not least of which because of the range of programming languages and tools that can be used with it - ...
Robots and getting computers to work with the physical world is fun; this blog looks at my own personal experimenting and building in this area.
Thursday, 4 April 2024
Top posts on this blog in March 2024
Wednesday, 26 July 2023
ChatGPT, Data Scientist - fitting it a bit
I apologize for the inconvenience, there seems to have been a technical issue with the code execution. Let's try to load the dataset again.
python# Load the new dataset
data_new = pd.read_csv('/mnt/data/papervcitation.csv')
# Display the first few rows of the dataset
data_new.head()
---------------------------------------------------------------------------
NameError Traceback (most recent call last)
Cell In[1], line 2
1 # Load the new dataset
----> 2 data_new = pd.read_csv('/mnt/data/papervcitation.csv')
4 # Display the first few rows of the dataset
5 data_new.head()
NameError: name 'pd' is not defined
Apologies for the inconvenience. It seems there was a mistake in the code execution. We need to import the pandas library first. Let's correct that and proceed.
pythonimport pandas as pd
# Load the new dataset
data_new = pd.read_csv('/mnt/data/papervcitation.csv')
# Display the first few rows of the dataset
data_new.head()
year citations no of papers
0 1999 5 2
1 2000 0 0
2 2001 23 3
3 2002 76 2
4 2003 36 4
- 'year': This seems to represent the year in which the papers were published or the citations were received.
- 'citations': This likely represents the total number of citations received in each year.
- 'no of papers': This probably represents the number of papers published in each year.
- The data spans 25 years, from 1999 to 2023.
- The average number of citations per year is approximately 23.56, with a standard deviation of about 24.63.
- The number of citations received in a year ranges from 0 to 81.
- The average number of papers published per year is approximately 4.96, with a standard deviation of about 4.29.
- The number of papers published in a year ranges from 0 to 14.
This dataset contains three columns: 'year', 'citations', and 'no of papers'.
Let's proceed with some basic data analysis. We'll start by checking the basic statistics of the dataset.
python# Check basic statistics of the data
data_new.describe()
year citations no of papers
count 25.000000 25.000000 25.000000
mean 2011.000000 23.560000 4.960000
std 7.359801 24.627356 4.286413
min 1999.000000 0.000000 0.000000
25% 2005.000000 2.000000 2.000000
50% 2011.000000 19.000000 4.000000
75% 2017.000000 39.000000 7.000000
max 2023.000000 81.000000 14.000000
Here are some basic statistics for the 'year', 'citations', and 'no of papers' columns:
Next, let's visualize the trend of 'citations' and 'no of papers' over the years. We'll also perform linear and polynomial fitting to these trends.
pythonimport numpy as np
from sklearn.linear_model import LinearRegression
from sklearn.preprocessing import PolynomialFeatures
# Create a linear regression model
linear_regressor = LinearRegression()
# Fit the linear regression model to the data
X = data_new['year'].values.reshape(-1,1)
y_citations = data_new['citations'].values
y_papers = data_new['no of papers'].values
linear_regressor.fit(X, y_citations)
y_citations_pred = linear_regressor.predict(X)
linear_regressor.fit(X, y_papers)
y_papers_pred = linear_regressor.predict(X)
# Fit a polynomial of degree 3 to the data
poly = PolynomialFeatures(degree=3)
X_poly = poly.fit_transform(X)
poly_regressor = LinearRegression()
poly_regressor.fit(X_poly, y_citations)
y_citations_poly_pred = poly_regressor.predict(X_poly)
poly_regressor.fit(X_poly, y_papers)
y_papers_poly_pred = poly_regressor.predict(X_poly)
# Plot the data and the fitted lines
plt.figure(figsize=(12,6))
# Plot the original data
plt.scatter(data_new['year'], data_new['citations'], color = 'blue', label='Citations')
plt.scatter(data_new['year'], data_new['no of papers'], color = 'red', label='No of Papers')
# Plot the linear fits
plt.plot(data_new['year'], y_citations_pred, color = 'blue', linestyle = '--', label='Citations Linear Fit')
plt.plot(data_new['year'], y_papers_pred, color = 'red', linestyle = '--', label='No of Papers Linear Fit')
# Plot the polynomial fits
plt.plot(data_new['year'], y_citations_poly_pred, color = 'blue', linestyle = ':', label='Citations Polynomial Fit')
plt.plot(data_new['year'], y_papers_poly_pred, color = 'red', linestyle = ':', label='No of Papers Polynomial Fit')
plt.title('Citations and No of Papers over Years with Linear and Polynomial Fits')
plt.xlabel('Year')
plt.ylabel('Count')
plt.legend()
plt.grid(True)
plt.show()
---------------------------------------------------------------------------
NameError Traceback (most recent call last)
Cell In[4], line 31
28 y_papers_poly_pred = poly_regressor.predict(X_poly)
30 # Plot the data and the fitted lines
---> 31 plt.figure(figsize=(12,6))
33 # Plot the original data
34 plt.scatter(data_new['year'], data_new['citations'], color = 'blue', label='Citations')
NameError: name 'plt' is not defined
I apologize for the oversight. It seems I forgot to import the matplotlib library which is used for creating plots. Let's correct that and proceed.
pythonimport matplotlib.pyplot as plt
# Plot the data and the fitted lines
plt.figure(figsize=(12,6))
# Plot the original data
plt.scatter(data_new['year'], data_new['citations'], color = 'blue', label='Citations')
plt.scatter(data_new['year'], data_new['no of papers'], color = 'red', label='No of Papers')
# Plot the linear fits
plt.plot(data_new['year'], y_citations_pred, color = 'blue', linestyle = '--', label='Citations Linear Fit')
plt.plot(data_new['year'], y_papers_pred, color = 'red', linestyle = '--', label='No of Papers Linear Fit')
# Plot the polynomial fits
plt.plot(data_new['year'], y_citations_poly_pred, color = 'blue', linestyle = ':', label='Citations Polynomial Fit')
plt.plot(data_new['year'], y_papers_poly_pred, color = 'red', linestyle = ':', label='No of Papers Polynomial Fit')
plt.title('Citations and No of Papers over Years with Linear and Polynomial Fits')
plt.xlabel('Year')
plt.ylabel('Count')
plt.legend()
plt.grid(True)
plt.show()
The plot above shows the original data for 'Citations' and 'No of Papers' along with the fitted linear and polynomial curves for each.
The linear fit provides a simple model of the overall trend, while the polynomial fit can capture more complex patterns in the data. However, the polynomial fit can also be more susceptible to fluctuations in the data and may not generalize as well to future data.
From the graph, it's evident that both the number of papers and citations have a general upward trend over the years. The number of citations seems to have a sharper increase compared to the number of papers, indicating that each paper might be getting more citations over the years. The polynomial fit captures the fluctuation in the data more accurately than the linear fit.
If you have more specific questions or if there's another aspect of the data you'd like to explore, please let me know!
Who wants to produce AI produced cartoon strips
Question: How easy is it produce a comic/cartoon using genetative AI? Let's start with using ChatGPT4o to produce cartoons. The idea wa...
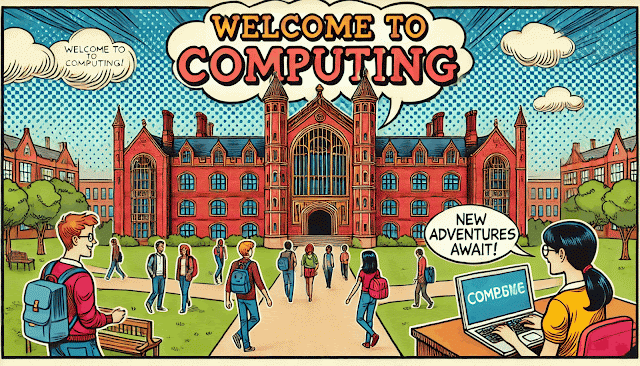